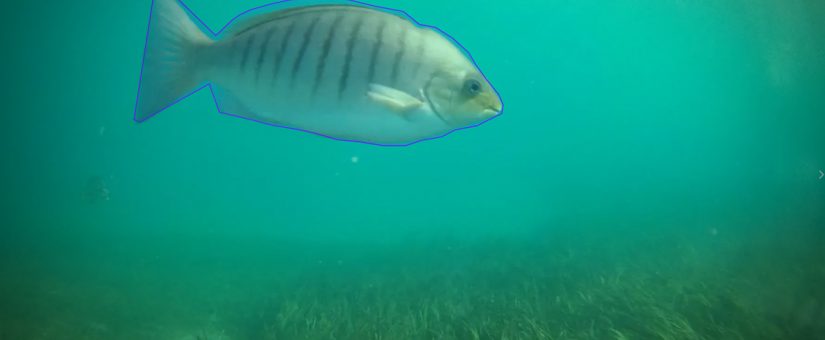
An annotated fish dataset in unconstrained seagrass habitats for machine learning algorithms
- Posted by Natasha Watson
- On March 17, 2021
By Ellen Ditria
A new data report and a publicly available dataset will allow further exploration of the use of computer vision techniques in aquatic environments.
Computer vision techniques in ecology have gained much attention as they can quickly and accurately process images from videos. They allow scientists to monitor both individuals and populations at unprecedented spatial and temporal scales.
This new dataset contains footage from remote underwater video (RUV) recordings of two common fish species, luderick (Girella tricuspidata) and Australian bream (Acanthopagrus australis) from seagrass habitats in the estuaries of two river systems in southeast Queensland, Australia
Applications of deep learning techniques into marine environments have shown promising results as a viable alternative for manual analysis, however annotating datasets requires considerable effort. Therefore, there is a need for accessible, quality annotated datasets for deep learning models to further the progress of applying these techniques in ecology. Here we provide a pre-annotated dataset to be used for a number of deep learning applications
The contributions of this dataset include:
(1) a comprehensive dataset of ecologically important fish species that captures the complexity of backgrounds observed in unconstrained seagrass ecosystems to form a robust and flexible model,
(2) a variety of modalities for rapid and flexible testing or comparison of different frameworks, and
(3) unaltered imagery for investigation of possible data augmentation and performance enhancement using pre and post-processing techniques.
Example frames demonstrating the variety of conditions including water clarity and differing camera angles.
Suggested applications
Specifically the dataset consists of 4,281 images and 9,429 annotations (9,304 luderick, 125 bream) at the standard high resolution (1920 × 1080 p).
Example frame of annotated Luderick using polygon segmentation masks.
Author recommendations for future work using this dataset include:
- Investigating pre- and post-processing steps to further examine the effects on performance.
- Comparing and testing new deep learning architectures.
- Creating multi species models by adding to other datasets.
- Investigating how water clarity or occlusion affects model performance.
Ongoing testing of this standardised dataset will be valuable in exploring the application of deep learning in aquatic systems.
0 Comments