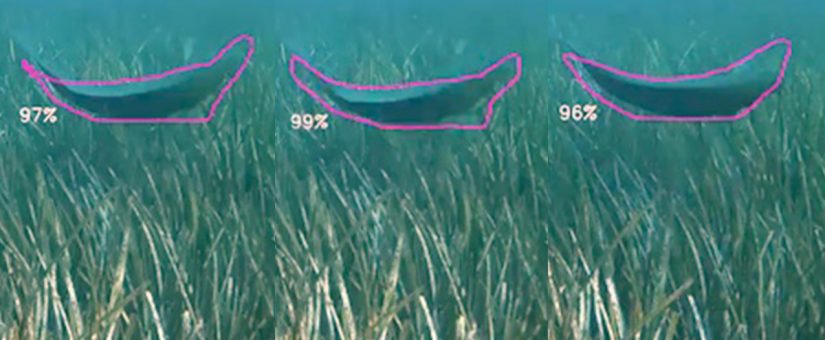
Deep learning to detect animal behaviour
- Posted by Natasha Watson
- On June 9, 2021
By Ellen Ditria
Studying and quantifying animal behaviour is important to understand how animals interact with their environments. However manually extracting and analysing behavioural data from large volumes of camera footage is often time consuming. Our new research shows how artificial intelligence can be a valuable tool to analyse underwater footage more effectively.
Deep learning techniques have emerged as useful tools in automating the analysis of certain behaviours under controlled or laboratory conditions. The complexities of using raw footage from the field however has resulted in this technology remaining largely unexplored as a possible data analysis alternative for animals in situ.
Counting the frequency of certain animal behaviours can be used as an important metric in understanding more complex behaviours. For example, kangaroos will stand on their hind legs with the head and ears raised, a behaviour frequently use by biologists to score vigilance to a perceived threat. The more times the animal exhibits the behaviour, the higher the vigilance score.
Using machine learning to automate these observational methods could assist in observer bias. It could also increase the sample size of studies when coupled with remote sensing methods like camera traps or remote video footage to collect data. Additionally, it may also limit confounding factors such as the observer’s presence that may affect the animal’s behaviour.
We found that by combining dense optical flow and deep learning algorithms, we can automatically identify the frequency of the grazing behaviour exhibited by Luderick (Girella tricuspidata) collected by underwater cameras.
Dense optical flow is able to estimate motion of pixels between two frames. The red-green-blue (RGB) coloured output denotes area and direction of pixel movement, while the black areas indicate no movement. The output is classified by the deep learning algorithm as “grazing” or “no grazing” behaviours. Additionally, we applied a spatio-temporal algorithm which looks at frames together, instead of in isolation, to see patterns in the pixel movement across frames.
Using this method across 18 videos we were able to detect 34 out of 37 grazing events (a rate of 92%).
Deep learning shows promise as a viable tool for determining animal behaviour from underwater videos. With further development it offers an alternative to current time-consuming manual methods of data extraction.
0 Comments