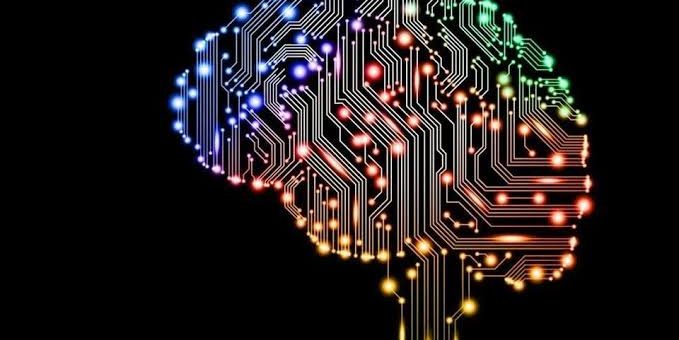
Integrating Artificial Intelligence and Citizen Science can Supercharge Ecological Monitoring
- Posted by Marina Richardson
- On October 19, 2020
Integrating Artificial Intelligence and Citizen Science can Supercharge Ecological Monitoring
By Dr. Eva McClure
People often imagine the future of technology, and science fiction has depicted many dystopian futures where artificial intelligence (AI) has taken over human civilisation. While AI surpassing human cognition is still in the realms of science fiction, AI technology and software with the capacity to perform tasks otherwise requiring human intelligence are being harnessed across many disciplines. In ecology, AI is becoming increasingly sort-after, but we are yet to realise its full potential.
Ecological monitoring is seeing a rapid uptake of AI and citizen science, scientific projects that engage volunteers of varying levels of expertise. These techniques allow scientists to collect and process volumes of data that were not previously possible using conventional methods. Ecological monitoring is integral to environmental management and biological conservation, but with advances in technology and the ability to collect big data sets, data processing has become a major bottleneck that requires novel solutions.
Until recently, citizen science and AI have been applied mostly in isolation. Our feature perspective highlights that integrating the computer power of AI with the people power of citizen science for collection and processing of large data sets can supercharge outcomes for ecological monitoring and conservation action.
Example of beneficial outcomes from integrating citizen science (CS) and artificial intelligence (AI), such as improved cost-effectiveness, data processing, accuracy, and stakeholder engagement, resulting in more efficient monitoring and improved conservation actions.
One such example of integrated AI-CS approaches is eBird, one of the world’s largest biodiversity-related citizen science projects, engaging >350,000 participants to gather data on >10,000 bird species. eBird has created a Human/Computer Learning Network to allow for the exchange of feedback and active learning between humans and machines for bird identification, to improve data quality for improved conservation and research outcomes.
AI is not yet renowned for serendipitous discovery in ecology, but unsupervised ‘deep learning’ may hold potential. Deep learning algorithms can automatically learn, extract features, and predict from data and so are becoming sought-after in monitoring to process raw images, videos, and audio. While the use of AI for these processes could conceivably replace the need for humans in ecological monitoring, there is intrinsic benefit in maintaining a human connection through citizen science involvement, such as enhancing the relationship between the public and the natural world.
So how does one decide whether to implement citizen science, AI, or both, for ecological monitoring? Managers of large ecological monitoring projects often have little guidance on which technique best suits their resource capacity and objectives. Based on current literature, we highlight key project attributes for consideration by project managers, to help guide these decisions.
Project attributes placed towards either citizen science (CS) or artificial intelligence (AI) indicate relatively higher importance for that technique, but not a lack of importance for the other technique. Project attributes placed in the middle indicate equally high importance for both CS and AI. All project attributes would be important to consider for integrated approaches.
Our study highlights that contrary to the popular human vs machine science fiction theme, there can be great benefits in the integration of citizen science and AI for improving methods in ecological monitoring, enhancing understanding of the natural world, and promoting positive outcomes for environmental management.
0 Comments