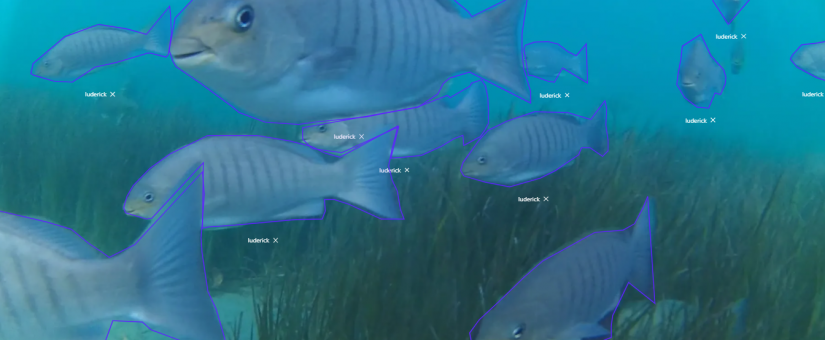
Deep learning for ecological monitoring: performance in novel habitats and benefits of varied training data
- Posted by Ellen Ditria
- On October 26, 2020
By Ellen Ditria, PhD candidate
Deep learning has fast become recognised as a powerful data processing tool for ecologists faced with vast amounts of image-based data. The ability of deep learning to accurately detect target species in videos and images unlocks the potential for rapid processing of data that usually requires hours of manual labour.
Despite these advantages, one of the key challenges is creating a model that can detect a given target in any situation. For example, accurately detecting a fish species from within different habitat types. This issue is known as domain shift; where the data the model has been trained on (e.g. seagrass habitats) is not an accurate enough representation of the data it is asked to predict on (e.g. reef habitats).
While we’ve previously shown that models trained on one seagrass habitat work on another seagrass habitat in a different location, most fish species utilise a range of different habitat types throughout their life. If you are monitoring a species that has a large distribution or frequents a number of different habitat types or ecosystems, will a model with limited variation in habitat type be enough?
We wanted to know:
- If a deep learning model trained in one habitat type (or ecosystem) can perform accurately on another habitat type that is visually dissimilar?
- If a model trained on both habitat types reduces the accuracy to detect fish in one habitat compared to a model that is trained on that habitat (eg seagrass trained model to seagrass test)
- In this scenario, would you need to create a different model for each new habitat type?
For our two habitat types, the “domain” can be quite different:
We found that models trained on a singular habitat, then tested on the other habitat do not perform well, demonstrating the phenomenon of ‘domain shift’.
However, when a combination of both habitats are used to train the model (3 separate models used with a randomized combination of training data), the resulting accuracy is high when compared to singularly trained models, with the performance accuracy of detection fish within a single frame being within 1% and accuracy of detecting the MaxN of a species within 2%
We conclude that if a researcher is interested in only a single habitat type, their model would be most accurate trained only on that habitat. No surprises there!
On the other hand, if researchers are interested in a range of habitats, we show that models trained on multiple habitats do not significantly reduce the quality or accuracy of the model’s ability to detect fish.
If a large distribution must be monitored, researched could use a combined model and continuously add to this model instead of re-training or creating new models for each habitat.
Full publication here: https://doi.org/10.1007/s10661-020-08653-z
0 Comments